1970-01-01 00:00:00
Welcome!
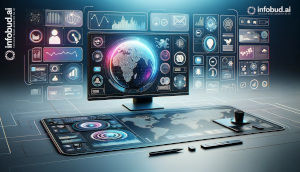
infobud.news is an AI-driven news aggregator that simplifies global news, offering customizable feeds in all languages for tailored insights into tech, finance, politics, and more. It provides precise, relevant news updates, overcoming conventional search tool limitations. Due to the diversity of news sources, it provides precise and relevant news updates, focusing entirely on the facts without influencing opinion. Read moreExpand